Quick show of hands: Who watched Groundhog Day for the umpteenth time last month?
Most viewers will tell you that it’s a story of a man who winds up living the same day over and over again. However, we prefer to think of it as the story of a man who ultimately learns how to live that same day – and ultimately life – better.
That teachable movie moment came to mind when we were tasked with addressing the central question of this month’s Viewpoint: What’s new in analytics?
“At first, we were tempted to take a pass,” says AFS Chief Analytics Officer, Mingshu Bates. “After all, many of the tools and practices that are making headlines and inspiring countless panel discussions today aren’t really new at all. In fact, most of them have been around for decades.”
“Then we realized that would be selling the subject short – because there’s actually a great deal that’s changed in terms of the way these tools are being adopted and applied. And that’s opening up a whole new world of issues and opportunities that today’s supply chain professionals really need to know about.”
We recently sat down with her to learn more.
Your statement about there not being much new in analytics surprised us. We would have thought that you’d be itching to acquaint us with the wonders of generative AI.
Considering that generative AI is older than many of our readers, that wouldn’t be accurate. In fact, it was introduced in the 1960s.
What is new is the recent excitement about it. In less than 18 months, we’ve essentially gone from watching Chat GPT make generative AI readily accessible to the general public, to witnessing smarter, more fluent variations being rolled out all the time. And we’ve seen many more businesses become interested in using it.
Obviously this has made many professionals’ (including analysts’) lives easier. Has it driven any other changes?
Lately, we’ve begun to see a rise in concerns about privacy, ethics and the risks of unintentional AI-related oversharing: Just as anything that you type into a large language model can be used to ask questions and find answers, any query or suggestion you type into a generative AI program can also be used to answer similar questions that are posed by anyone else, including – quite possibly – your competitors.
These concerns have led more companies to pursue the development of their own exclusive-use large language models, a prospect that can be both expensive and time-consuming.
Has generative AI helped with data democratization?
Great question – especially since finding ways to enable more employees to access data and make data-driven decisions (even if they aren’t professional data scientists or IT professionals) has recently become a huge hot button for many companies.
The short answer is yes, at least to a degree. It’s undoubtedly made a lot of additional data readily available to the average user, which is essentially what data democratization is all about. However, data availability isn’t automatically synonymous with data accuracy, so it can also complicate things.
Tell us more. . .
Have you ever seen a variation of this popular Abraham Lincoln meme?
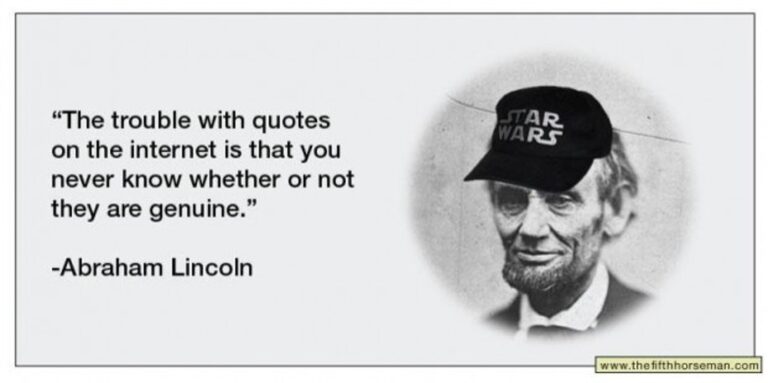
It pretty much sums up the challenge that today’s businesses face when pursuing data democratization: Even though the average employee can now access lots of data with the help highly advanced tools like generative AI, there’s always the possibility that the data they uncover – no matter how legitimate it appears – isn’t valid.
Granted, this isn’t only a challenge because of generative AI; employees can pull inaccurate data from anywhere, even password-protected company portals with extensive indexes and data libraries. However, generative AI has definitely exacerbated the problem.
Is there a solution or practice that’s trending as a result of this?
Smart companies are placing more emphasis on cleaning up and standardizing every data point members of their organizations are using – bearing in mind that the average employee doesn’t necessarily have the ability to differentiate between information that’s inaccurate, redundant or outdated and information that’s been fully vetted, thoroughly fact-checked and properly synthesized.
Sounds like a tall order.
It is, which is why some companies are opting to outsource some or all of their data management and validation instead. Ultimately, this has accelerated the growth of Analytics as a Service (AaaS).
We’ve spent a fair amount of time talking about an analytics tool that’s almost old enough to draw a social security check. Is there – perhaps – something a bit newer we could discuss?
How about edge computing? It’s only been around since the 1990s.
Which makes it younger, although not truly young.
Regardless, it’s another analytical tool that has recently been gaining a lot of traction.
Depending on which source you reference, it was anywhere from a $16 billion – $53 billion market by the end of last year. And it’s expected to have grown more than tenfold by the 2030s.
Those are huge variations in numbers.
It’s a prime example of why companies need to have experienced data analysts on their payroll – or if they outsource their data analysis, have their provider on speed dial. Because sometimes it takes a professional to figure out which pieces of data to apply and what weight to assign them.
What’s responsible for the surge in edge computing?
The desire to apply edge more often has always been there, especially in certain business functions like the supply chain. And some industries, like healthcare, managed to embrace it fairly quickly.
But now the bandwidth and capability are there for many other industries and local locations, thanks largely in part to the growing computer power that’s present in edge computing technologies and the advent of 5G networks.
So how is it being applied?
You name it. Parcel carriers are using it to redirect packages while they’re in transit. Warehouse operators are using it to enable robotic devices to maneuver safely around their distribution centers. Carriers with self-driving trucks are using it to help these vehicles operate safely based on current traffic conditions.
The possibilities really are endless.
Sounds like a topic for a completely different Viewpoint column.
All of these topics are – and so is reminding businesses that when it comes to solid analytics, it’s not always about new and different but about staying abreast of the latest advanced analytics trends – and to mindfully consider how and when to embrace them at their companies. It’s equally important not to overlook the continuing importance of applying traditional analytics tools, too.
In other words, you’re not ready to toss your old analytical toolbox out just yet?
Precisely. In fact, as Ned Ryerson from Groundhog Day might say, some of those traditional tools are still doozies!